In This Story
In the rapidly evolving field of cybersecurity, the integration of generative AI and large language models (LLMs) is a game-changer. While offering immense potential for positive applications, these tools also pose significant risks if misused. Mohamed Gebril, an associate professor at the Department of Cyber Security Engineering at George Mason University, is spearheading a project to use generative AI and LLMs to better identify the very threats they pose.
The project is a collaborative effort between George Mason and the Virginia Military Institute (VMI) funded by the Commonwealth Cyber Initiative Northern Virginia Node. Gebril, along with VMI's Sherif Abdelhamid, is assembling a team including master's and undergraduate students to assist with research and is preparing educational workshops to introduce high school and middle school students to the research topic. Gebril’s approach aims to equip future cybersecurity professionals with the knowledge and skills needed to tackle emerging threats.
Automating threat detection
The initiative aims to leverage the power of AI to enhance threat detection and response mechanisms, ultimately making cybersecurity operations more efficient and effective. One of the primary advantages of using AI in cybersecurity is its ability to automate processes that were traditionally manual and time-consuming. Harnessing the beneficial aspects of generative AI for threat-hunting operations involves detecting malicious activities and monitoring data logs in real-time.
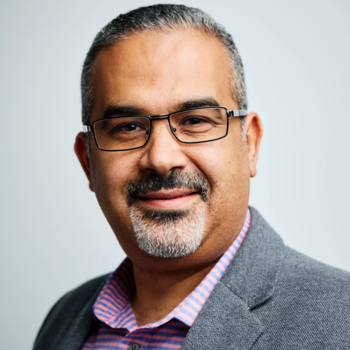
"AI has been very helpful in automating this process instead of doing it manually,” Gebril explained. “It can generate the alerts, automate the notifications, and make the instant response.” By automating these tasks, organizations can respond to threats more quickly and efficiently, reducing the potential damage caused by cyberattacks.
Preparing for prompt-injection attacks
A significant challenge in the realm of AI-driven cybersecurity is the threat of prompt-injection attacks, which involve malicious actors using AI prompts to generate harmful outputs, such as malware, said Gebril. His core objective is to create mechanisms for detecting malicious intent, particularly in prompts that are subtle and indirect.
"What we're hoping to get out of this project is to be able to develop a novel method, a novel mechanism, to detect such malicious intent that is meant to be used or developed by indirect prompt injection attacks," Gebril said. This involves using advanced AI techniques, such as fuzzy reasoning and deep learning, to analyze and interpret data in real-time.
By leveraging the power of AI, Gebril and his team are working to create more robust and effective threat detection systems, ultimately contributing to a safer digital landscape.